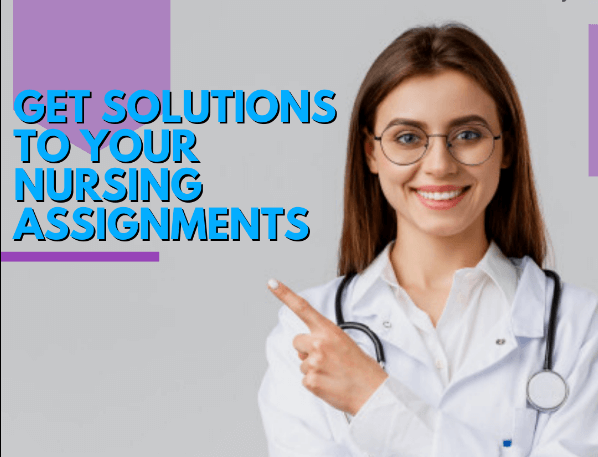
Analysis and Evaluation of Individual and Population Health Data
Introduction
In the field of healthcare, the availability and analysis of individual and population health data play a vital role in understanding the overall health status of individuals and communities. NR.210.817 refers to the analysis and evaluation of individual and population health data, which involves various techniques and methodologies to extract meaningful insights and inform decision-making processes. This article aims to provide a comprehensive analysis and evaluation of NR.210.817, highlighting its importance, key components, methods, and the impact it has on improving healthcare outcomes.
Importance of NR.210.817
NR.210.817 holds significant importance in healthcare as it allows for a comprehensive assessment of individual and population health data. By analyzing and evaluating this data, healthcare professionals can gain valuable insights into disease patterns, risk factors, treatment effectiveness, and the overall health status of individuals and communities. This information serves as a foundation for evidence-based decision-making, policy formulation, resource allocation, and the development of targeted interventions to address health issues effectively.
Key Components of NR.210.817
NR.210.817 encompasses several key components that are crucial for the analysis and evaluation of individual and population health data.
These components include:
Need Help Writing an Essay?
Tell us about your ASSIGNMENT and we will find the best WRITER for your paper.
Get Help Now!- Data Collection: The first step in the process is the collection of individual and population health data. This can be done through various sources such as electronic health records, surveys, registries, and public health surveillance systems. The data collected may include demographic information, medical history, laboratory results, lifestyle factors, and socio-economic indicators.
- Data Cleaning and Preparation: Once the data is collected, it undergoes a cleaning and preparation process. This involves removing any errors, inconsistencies, or missing values to ensure the accuracy and reliability of the data. Data transformation and standardization techniques may also be applied to facilitate analysis and comparison across different datasets.
- Data Analysis: After data cleaning and preparation, various statistical and analytical techniques are applied to extract meaningful insights from the data. This may involve descriptive analysis to summarize the characteristics of the data, inferential analysis to make predictions or draw conclusions, and exploratory analysis to identify patterns or associations.
- Data Visualization: Data visualization techniques are used to present the findings in a visual format, such as graphs, charts, and maps. Visual representations make it easier to interpret complex information and communicate key findings to stakeholders and decision-makers.
- Data Interpretation: Once the data is analyzed and visualized, it needs to be interpreted in the context of the research question or problem being addressed. This involves drawing conclusions, identifying trends or patterns, and making evidence-based recommendations.
- Evaluation of Data Quality: Evaluating the quality of individual and population health data is essential to ensure its reliability and validity. This evaluation includes assessing data completeness, accuracy, timeliness, and consistency. Validating the data against external sources or conducting data audits can help identify any potential biases or errors.
Methods for Analysis and Evaluation
NR.210.817 employs various methods for the analysis and evaluation of individual and population health data.
Some commonly used methods include:
- Descriptive Statistics: Descriptive statistics summarize and describe the main features of the data, such as measures of central tendency (mean, median, mode) and measures of dispersion (variance, standard deviation). These statistics provide a snapshot of the data’s characteristics and help in understanding the distribution of variables.
- Inferential Statistics: Inferential statistics are used to make predictions or draw conclusions about a population based on a sample. Techniques like hypothesis testing, regression analysis, and correlation analysis are commonly employed to infer relationships and associations between variables.
- Epidemiological Studies: Epidemiological studies play a crucial role in analyzing and evaluating population health data. These studies investigate the distribution and determinants of health-related events in populations. They help identify risk factors, study disease patterns, and evaluate the effectiveness of interventions.
- Data Mining and Machine Learning: Data mining and machine learning techniques are utilized to uncover hidden patterns or relationships within large datasets. These methods can identify predictive models, classify patients into different risk categories, and support clinical decision-making.
- Geospatial Analysis: Geospatial analysis combines geographic information systems (GIS) with health data to examine spatial patterns and relationships. It helps identify hotspots of disease prevalence, access to healthcare services, and environmental factors that influence health outcomes.
Impact on Healthcare Outcomes
The analysis and evaluation of individual and population health data have a significant impact on healthcare outcomes.
NR.210.813 Complexity of Health Care Systems
By leveraging NR.210.817, healthcare professionals can:
- Improve Patient Care: By analyzing individual health data, healthcare providers can identify personalized treatment options and interventions. They can monitor patient progress, identify potential risks, and make evidence-based decisions to improve health outcomes.
- Public Health Interventions: Population health data analysis enables public health officials to identify health trends, risk factors, and disease patterns at the community or population level. This information helps in designing targeted interventions, health promotion campaigns, and preventive measures to reduce the burden of diseases.
- Health Policy Development: NR.210.817 informs health policy development by providing policymakers with accurate and timely information about the health status of populations. It helps in identifying priority areas, setting health targets, and allocating resources effectively.
- Healthcare Resource Allocation: The analysis of population health data assists in identifying healthcare resource gaps and allocating resources based on the identified needs. It allows healthcare systems to optimize resource allocation, reduce inefficiencies, and improve access to care.
- Research and Innovation: Individual and population health data analysis provides a foundation for research and innovation in healthcare. Researchers can use this data to identify research gaps, generate hypotheses, and evaluate the effectiveness of new interventions or therapies.
Conclusion
NR.210.817 plays a vital role in the analysis and evaluation of individual and population health data. It provides healthcare professionals with valuable insights into disease patterns, risk factors, and treatment effectiveness. By employing various methods and techniques, NR.210.817 helps improve patient care, inform public health interventions, support policy development, optimize resource allocation, and drive research and innovation. Embracing NR.210.817 is crucial for achieving evidence-based decision-making and improving healthcare outcomes.
Get Fast Writing Help – No Plagiarism Guarantee!
Need assistance with your writing? Look no further! Our team of skilled writers is prepared to provide you with prompt writing help. Rest assured, your work will be entirely original and free from any plagiarism, as we offer a guarantee against it. Experience swift and dependable writing assistance by reaching out to us today!
Top-Rated Essay Writing Service | GET Flawless papers for All Your classes!
